Blockchain in Healthcare: Real-World Case Studies and Implementations – Web3oclock
Table of Contents:
Role of Blockchain in Healthcare
Benefits of Using Blockchain in Healthcare
Case Studies & Implementations of Blockchain in Healthcare
Challenges & Limitations of Using Blockchain in Healthcare
Today, one of the biggest challenges in healthcare is managing patient data securely and efficiently. From the medical reports to unauthorized access, the risks remain high. Blockchain technology, best known for powering digital cryptocurrencies, has come aboard to help solve these issues in healthcare. By providing safe and decentralized storage, Blockchain aims to provide a transparent and secure healthcare system. So, how does it work, and what impact does Blockchain have on healthcare? In this blog, we will explore how Blockchain technology is bringing transparency, security, and efficiency to the Healthcare system. Well, let’s break that down!
You might wonder, what role Blockchain is playing in this healthcare system. Why Blockchain technology has become so important in healthcare? Well, Blockchain in healthcare plays a transformative role in addressing the most important issues that revolve around data security, interoperability, transparency, and efficiency.
Role of Blockchain in Healthcare:
1. Enhanced Data Security:
In healthcare, Blockchain contributes to the security of sensitive health data. Health systems are a prime target for cyber-attacks, whereby data breaches lead to unauthorized access. Blockchain’s decentralized and cryptographic guaranteed that:
Immutable Data: The moment patient data enters the blockchain, it can no longer be tampered with or changed without the network consensus that ensures the integrity of the data.
Decentralized Access: Blockchain eliminates one single point of failure, making healthcare data less vulnerable to hacking.
Encryption: Patient data on the blockchain is encrypted, and only those subjected to the correct decryption key have access to information for privacy and confidentiality’s sake.
2. Enabling Interoperability:
Healthcare data typically resides in silos across various systems. This creates barriers to sharing patient information between providers. Blockchain provides a unified, decentralized platform for seamless data sharing:
Access Across Providers: Blockchain provides access and sharing of a patient’s data with different systems of healthcare providers, whether those are from hospitals, clinics, or specialists.
The Patient-Centric Model: Blockchain puts the patient in complete control of their data, giving patients discretion over who sees their information. This helps bring more transparency into health care.
Interoperable Systems: Blockchain helps integrate various healthcare systems where access to medical records, test results, and treatment plans can be viewed in real time for better care coordination.
3. Supply Chain Management Improvement:
In the field of pharmaceuticals, blockchain improves one critical aspect of traceability and transparency of drug supply. This is important in ensuring the authenticity of drugs and that counterfeit medications do not reach patients:
Traceability: Blockchain tracks every step of the drug manufacturing, distribution, and delivery process tamper-proof log of the drug’s journey.
Transparency: Both the manufacturers and distributors, pharmacies, and regulators within the supply chain can access the real-time data of drug batches, thus helping in building accountability.
Fraud Prevention: Blockchain prevents the entry of counterfeit drugs into market circulation since only authorized participants are granted access to the supply chain, fraudsters can be deterred.
4. Smoothening Medical Billing and Claims Processing:
Blockchain can ease the complex and time-consuming process of medical billing and insurance claims with its transparent, automated system:
Smart contracts: These self-executing contracts would automate the processes of payment between providers patients and insurance companies. Treatment is delivered, while smart contracts verify for coverage and allow for payment to be made.
Fraud Reduction: The clarity of blockchain means that all transactions are clear as crystal, not only transparent but also verifiable. This reduces fraudulent claims.
Efficiency: Elimination of intermediaries and automation of the verification process quickens up the reimbursement process due to reduced administrative overhead.
5. Improvement in Clinical Trials and Research:
Blockchain enhances the integrity, transparency, and security of clinical trials and research by providing a tamper-proof platform for data management in:
Data Integrity: Blockchain ensures that no alteration or manipulation in the clinical trial data, whether it involves patient recruitment, trial results, or consent forms, will occur and that all elements are accurate.
Management of Patient Consent: Blockchain documents and manages patient consent to trials in a very secure manner.
Data Sharing: Researchers can securely share data on trials with their collaborators and regulatory bodies, being sure that none of the intellectual property or patient privacy is breached.
6. Improve Public Health and Disease Surveillance:
Blockchain can play a very pivotal role in public health since it allows real-time sharing of data on the surveillance or outbreaks of diseases to manage them:
Real-Time Data Tracking: Blockchain enables the sharing of health data by different healthcare providers in real-time, public health authorities, and researchers in a secure manner, which will be very helpful in pandemics or outbreaks of some diseases. Vaccination and immunization information can be stored securely and managed through blockchain technology, whereby helps public health authorities to track immunization coverage and monitor the distribution of vaccines correspondingly.
Data Privacy: Blockchain anonymizes and protects sensitive health data while providing valuable insights into public health research and policymaking.
7. Telemedicine and Remote Monitoring:
The emergence of telemedicine and remote health monitoring devices leverage blockchain to ensure security and privacy in patients’ data during online consultations and the transfer of data:
Secure Data Transmission: The blockchain encrypts and secures patient data from wearables that may relate to health monitoring, such as glucose or heart rate monitors, making this type of information accurate and tamper-proof.
Privacy in Telemedicine: Blockchain protects the privacy of a patient in a telemedicine session. That ensures consultation and medical records stay private.
Patient Control: The patient can stay in control by determining who should get access to his health data and under what conditions.
8. Genomic Data Sharing:
Genomic information is sensitive and personal, still, the sharing of genomic data is very important. Blockchain can manage and share genomic data securely in the following manner:
Ownership: The ownership of genomic data rests with the patient. The right to decide upon who gets access to it and when, whenever necessary for research or other purposes, is solely left to the patient’s discretion.
Privacy and Security: Blockchain ensures that genomic data is stored and shared in a secure environment, reducing the potential incidents of access and hacking.
Monetization: Blockchain provides an avenue for an individual to share their genomic data with researchers or pharmaceutical companies at a prescribed fee while still having total control of use.
9. Fraud Prevention and Compliance:
Fraud in healthcare has been reduced because Blockchain will maintain a transparent and irreversible record of all transactions and activities:
Blockchain Prevents Fraudulent Claims: With blockchain technology, the transparency it provides to insurance companies means verifying each claim for validity to prevent fraud.
Regulation: Blockchain provides regulatory authorities with a transparently tamper-proof audit trail for proving the compliance of healthcare providers with industry standards and regulations.
10. Public Health Surveillance:
Blockchain can be used to collect, analyze, and share public health data securely, helping track the spread of diseases like COVID-19.
Real-Time Data Sharing: Blockchain can enable real-time sharing of health data between healthcare providers, researchers, and government bodies.
Privacy Protection: Blockchain ensures that personal health data is anonymized while still providing useful information for public health surveillance.
Outbreak Tracking: Blockchain can help track the spread of diseases and manage vaccination distribution more efficiently.
But what will be the benefits for you as a user? Blockchain offers significant benefits for patient data security and management by creating a system that is decentralized, immutable, and transparent.
Benefits of Using Blockchain in Healthcare:
1. Enhanced Data Security and Privacy:
Blockchain applies cryptographic methods in securing patient information. Once information about the patient is on the blockchain, the information is encrypted and stored in a decentralized network, where accessing and tampering will be very difficult for an unauthorized user. Each block of information is joined with its previous one in a cryptographic hash, where only authorized parties will have access to and decrypt the data.
Benefit: It helps to prevent patient information, such as medical records, test results, and other personal health information, from being accessed by cyber hackers through cyberattacks and data breaches. Furthermore, blockchain gets rid of any central storage systems as the technology does not necessitate nodes having to store data in one single location, and it reduces single points of failure that the hacker can use to obtain entry.
Example: Permissioned access would mean that in a blockchain-based healthcare system, only the allowed doctor, nurse, or healthcare provider will get to view a patient’s case history after permission from the patient. This implies data privacy and a very reduced chance of data theft.
2. Patient Control Over Data (Ownership and Consent Management):
The blockchain affords the patient ownership of their health data. Through the blockchain, a patient can manage their data by controlling who has access to those records. They may grant or revoke permission from healthcare providers, insurance companies, or even researchers to make certain that data is shared only upon explicit consent.
Benefit: In return, benefits come in the form of increased transparency and trust. It involves active participation by patients in managing their health data. It rules out the use of any third-party intermediary and central databases; this means access or sharing of the patient’s data cannot be done without prior permission.
Example: Patients can give temporary permission through blockchain to a new doctor to access the medical records for a particular treatment, and once the particular treatment is over, they can revoke the doctor’s permissions.
3. Immutable and Tamper-Proof Records:
One of the main characteristics of blockchain is its immutability. Once data has been added, it cannot be changed or deleted. Each time a healthcare professional edits a patient record by providing a new diagnosis, prescribing, or treatment, the information gets labeled with the time and date it is entered and gets added to the blockchain as a new block.
Benefit: This, in return, provides the benefit wherein medical records are tamper-proof. None can alter any patient information or trace history and change the prescription without going undetected. This is one of the most crucial features of ensuring that medical fraud, misdiagnosis, and data corruption cases are at complete avoidance.
Example: A blockchain-powered hospital management system will ensure that any modification made to a patient’s record is authenticated. In case of alteration of any earlier entry, the system will flag the change immediately, guaranteeing accountability and transparency.
4. Interoperability and Data Sharing:
Blockchain allows the secure sharing of a patient’s data amongst various healthcare providers and institutions. It creates a unified, decentralized system whereby, with need and authentic consent, various healthcare entities have access to updated medical records, lab reports, and other health data. Data stored on a blockchain is shareable right across systems, irrespective of the software or network in use by different organizations.
Benefit: Interoperability ensures access to patient records is readily available, thereby negating the delay in treatment or duplication of tests or procedures. Thus, healthcare professionals will be in a stronger position to coordinate better for better patient outcomes-assuring better coordination in the care experience.
Example: If a patient sees different doctors in various locations, through blockchain, their medical record updates could be performed instantly and at every location. This would avoid gaps in information and the incidence of medical errors due to miscommunication or outdated records.
5. Reduced Administrative Costs and Errors:
Blockchain automates patient data management and consent, eliminating paperwork, the need for manual data entry, and record checking. Further, smart contracts could provide complete automation of patient check-ins, billing, insurance claims processing, and consent, assuring that any business processes are executed per rules established upfront.
Benefit: This will reduce human errors while handling sensitive information about patients, and hence it will cut down on the costs associated with administration needed for health records maintenance. Since the blockchain cuts out the intermediaries, it speeds up workflows, cutting inefficiencies in the management of healthcare services, thereby helping a healthcare provider devote greater time to patient care.
Example: A hospital using blockchain can automate its insurance claim verification through smart contracts with the least possibility of errors and disputes. This cuts administrative overhead and ascertains that the patients get timely approvals for treatments.
Here are some real-world case studies and implementations of blockchain in healthcare, demonstrating how different organizations have utilized the technology to improve data security, transparency, and management in healthcare systems.
Case Studies & Implementations of Blockchain in Healthcare:
1. MedRec: Blockchain for Electronic Medical Records (EMR)
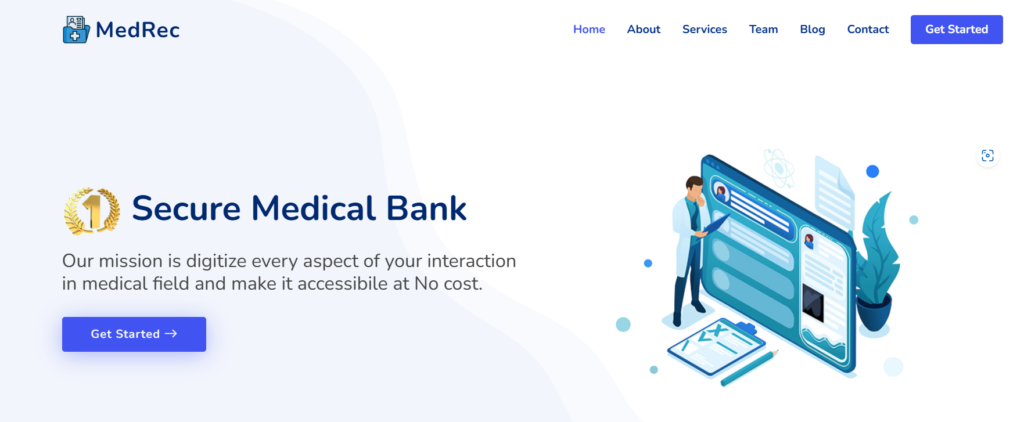
Use Case: Secure Management of Electronic Medical Records
Overview: Developed by MIT Media Lab, MedRec is a blockchain-based system for managing electronic medical records (EMRs). The platform aims to provide patients with a comprehensive, immutable medical history across multiple healthcare providers while ensuring data integrity and security.
Implementation: MedRec allows patients to access their medical history and manage consent for data sharing. Healthcare providers can securely share medical records, ensuring interoperability between different systems without compromising data privacy.
2. MedicalChain: Blockchain for Secure Health Record Sharing
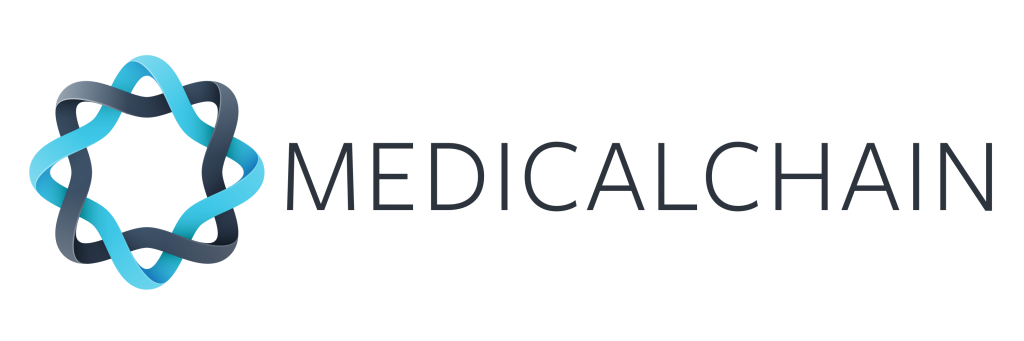
Source: medicalchain.com
Use Case: Secure Patient Data Sharing and Telemedicine
Overview: MedicalChain uses blockchain to create a secure platform where patients can store and share their medical records. The platform also offers telemedicine services, allowing doctors to consult with patients using verified medical data on the blockchain.
Implementation: Patients can create a comprehensive health record, stored on a blockchain that can be shared with healthcare professionals, labs, or insurance companies with explicit permission. Telemedicine consultations are recorded on the blockchain, ensuring accurate medical histories and treatment records.
3. Guardtime: Blockchain for Healthcare Data Integrity in Estonia
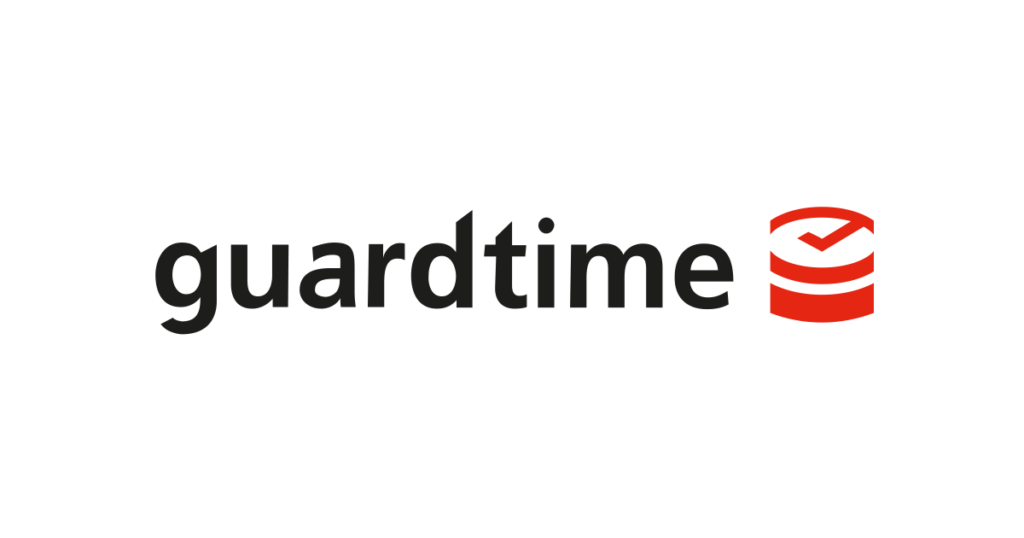
Source: Guardtime
Use Case: Ensuring Data Integrity for National Healthcare System
Overview: Estonia’s national healthcare system uses blockchain technology, provided by Guardtime, to secure healthcare data for its citizens. Estonia has been a global leader in implementing blockchain across various sectors, including healthcare, to enhance data security.
Implementation: Guardtime’s blockchain-based system stores digital signatures (hashes) of healthcare records on the blockchain, allowing verification of data authenticity and preventing tampering. Healthcare providers, patients, and regulatory authorities can verify the integrity of records without accessing the actual data.
4. Pharmaceutical Supply Chain: IBM and Walmart’s Blockchain Initiative
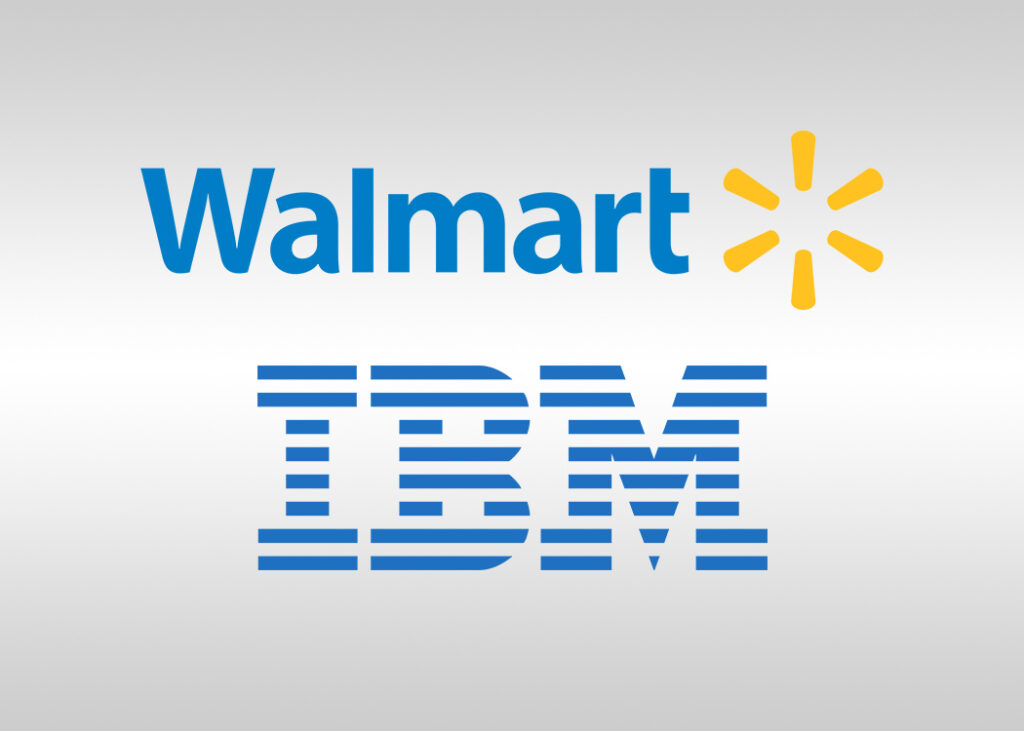
Source: bitexpert.io
Use Case: Drug Traceability and Counterfeit Prevention
Overview: IBM and Walmart teamed up to use blockchain to improve the transparency and traceability of pharmaceutical supply chains. The project aims to combat counterfeit drugs by tracking the entire lifecycle of medications.
Implementation: Using IBM’s blockchain platform, the project tracks every stage of the drug supply chain, from manufacturing to distribution to pharmacies. Each transaction is logged on the blockchain, ensuring that only authorized participants can update or access the data.
5. SimplyVital Health: Blockchain for Healthcare Payments and Data Sharing
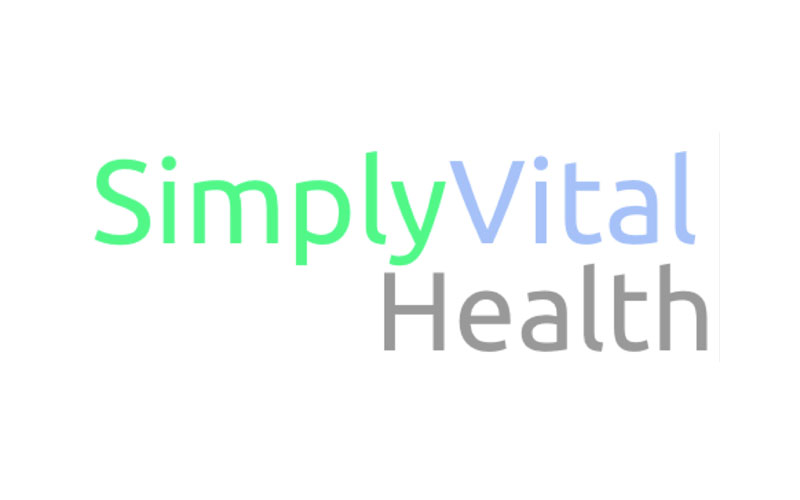
Source: Blocktribune.com
Use Case: Payment Transparency and Secure Data Sharing for Value-Based Healthcare
Overview: SimplyVital Health is a blockchain-based platform designed to facilitate value-based healthcare by providing secure data sharing and transparent payments between healthcare providers. The platform focuses on care coordination and tracking outcomes to align with healthcare reimbursement models based on patient outcomes.
Implementation: SimplyVital uses blockchain to allow healthcare providers to share medical data securely, enabling better collaboration for patient care. The platform also tracks payments and reimbursement transparently, ensuring that providers are paid based on patient outcomes, rather than service volume.
6. Solve. Care: Blockchain for Healthcare Administration
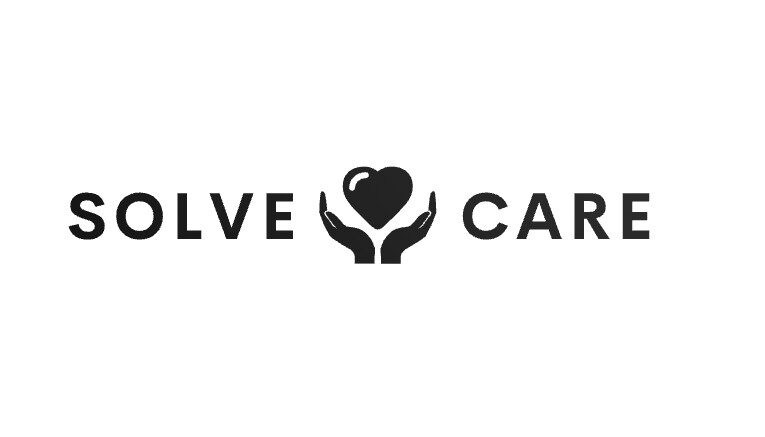
Source: ndsp.com.au
Use Case: Streamlining Healthcare Administration and Patient Payments
Overview: Solve. Care is a decentralized platform that uses blockchain to streamline healthcare administration, including appointment scheduling, medical billing, and patient payments. The platform leverages smart contracts to automate processes, reducing administrative overhead and improving transparency.
Implementation: Patients, providers, and insurance companies can interact on the blockchain, automating various administrative tasks. Smart contracts ensure that payments are made automatically once services are provided, reducing delays in billing and reimbursement.
7. BurstIQ: Blockchain for Precision Medicine and Data Sharing
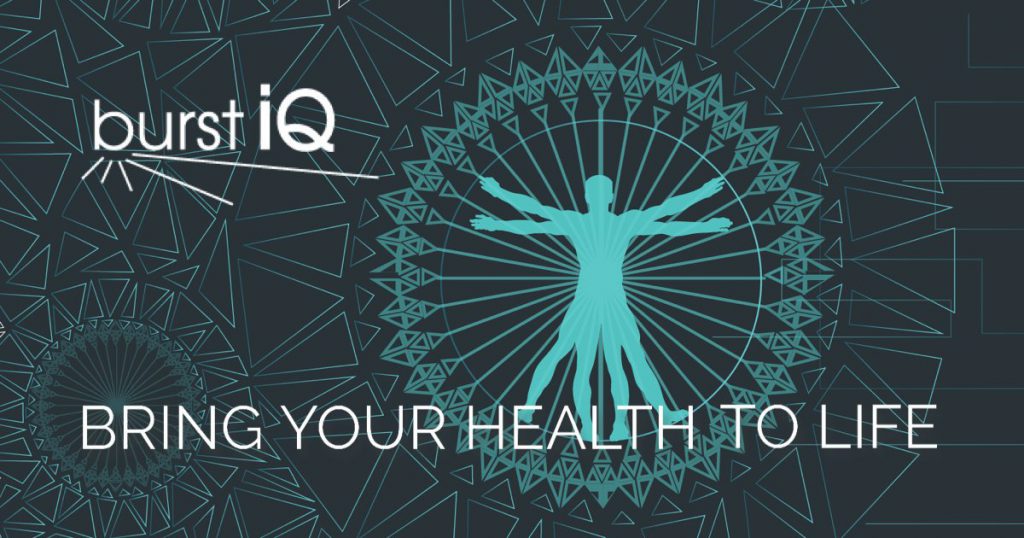
Source: blockchainmagazine.net
Use Case: Secure Data Exchange for Precision Medicine and Research
Overview: BurstIQ uses blockchain to securely share large datasets for precision medicine, enabling researchers, healthcare providers, and patients to exchange data without compromising privacy. The platform is designed to promote collaboration in medical research while ensuring data security.
Implementation: The platform stores and manages patient data on the blockchain, allowing it to be securely shared with authorized parties for research purposes. Patients retain control over their data, and they can choose to share it with specific researchers or healthcare providers.
But there are some challenges and limitations as well, which you need to be aware of.
Challenges & Limitations of Using Blockchain in Healthcare:
1. Adoption:
Integrating blockchain into existing healthcare systems requires significant infrastructure changes and regulatory support.
2. Scalability:
Blockchain networks can become slow and costly when handling large amounts of data, such as detailed medical records.
3. Data Privacy Concerns:
Despite its security features, the decentralized nature of blockchain raises concerns about how personal data is stored and shared across networks.
Blockchain technology has the potential to transform healthcare by improving the security, transparency, and efficiency of medical systems. While challenges remain, including scalability and regulatory hurdles, the technology offers promising solutions for managing patient data, securing drug supply chains, ensuring the integrity of clinical trials, and streamlining medical billing. As the technology matures, more healthcare organizations are likely to adopt blockchain, ultimately leading to better patient outcomes and more efficient healthcare systems.
But what does the future hold for blockchain in healthcare? How will it impact patient outcomes, medical research, and healthcare accessibility in the years to come? Could blockchain become the backbone of a fully digitized healthcare ecosystem?
For more insights and updates on blockchain, Web3, subscribe to our newsletter and stay informed!