DeepSeek has gained recognition in the AI community with its latest models, DeepSeek R1, DeepSeek V3, and DeepSeek R1-Zero. Each model offers unique capabilities and is designed to address different AI applications. DeepSeek R1 specializes in advanced reasoning tasks, employing reinforcement learning to improve logical problem-solving skills. Meanwhile, DeepSeek V3 is a scalable natural language processing (NLP) model, leveraging a Mixture-of-Experts (MoE) architecture to manage diverse tasks efficiently. On the other hand, DeepSeek R1-Zero takes a novel approach by relying entirely on reinforcement learning without supervised fine-tuning.
This guide provides a detailed comparison of these models, exploring their architectures, training methodologies, performance benchmarks, and practical implementations.
DeepSeek Models Overview
1. DeepSeek R1: Optimized for Advanced Reasoning
DeepSeek R1 integrates reinforcement learning techniques to handle complex reasoning. The model stands out in logical deduction, problem-solving, and structured reasoning tasks.
Real-World Example
Input: “In a family tree, if Mark is the father of Alice and Alice is the mother of Sam, what is Mark’s relation to Sam?”
Expected Output: “Mark is Sam’s grandfather.”
DeepSeek R1 efficiently processes logical structures, ensuring its responses are both coherent and accurate.
2. DeepSeek V3: General-Purpose NLP Model
DeepSeek V3, a versatile NLP model, operates using a Mixture-of-Experts (MoE) architecture. This approach allows the model to scale effectively while handling various applications such as customer service automation, content generation, and multilingual processing.
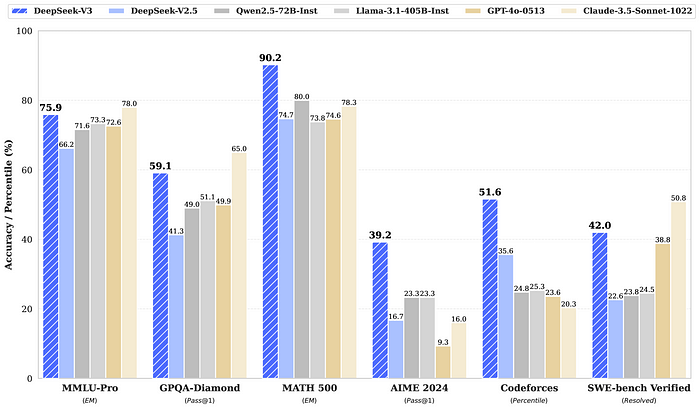
Real-World Example
DeepSeek V3 ensures that responses remain concise, informative, and well-structured, making it ideal for broad NLP applications.
3. DeepSeek R1-Zero: Reinforcement Learning Without Supervised Fine-Tuning
DeepSeek R1-Zero takes a unique approach. It is trained exclusively through reinforcement learning without relying on traditional supervised fine-tuning. While this method results in strong reasoning capabilities, the model may occasionally generate outputs that lack fluency and coherence.
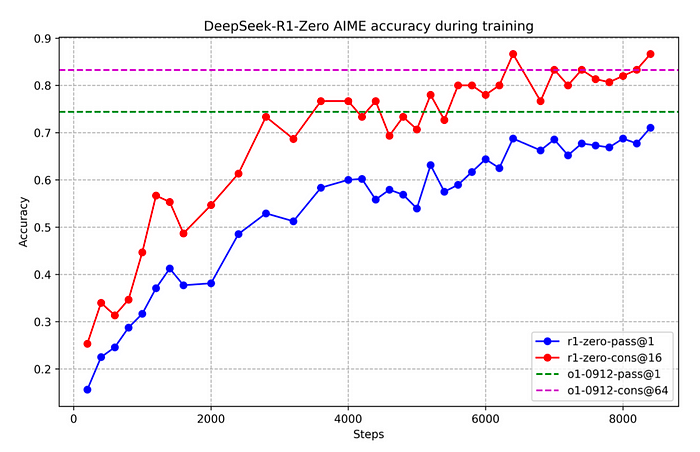
Real-World Example
Input: “Describe the process of volcanic eruption.”
Expected Output: “Volcanic eruptions occur when magma rises beneath the Earth’s crust due to intense heat and pressure. The magma reaches the surface through vents, causing an explosion of lava, ash, and gases.”
DeepSeek R1-Zero successfully conveys fundamental scientific concepts but sometimes lacks clarity or mixes language elements.
Model Architecture: How They Differ
1. DeepSeek V3’s Mixture-of-Experts (MoE) Architecture
The Mixture-of-Experts (MoE) architecture makes large language models (LLMs) more efficient by activating only a small portion of their parameters during inference. DeepSeek-V3 uses this approach to optimize both computing power and response time.
DeepSeek-V3 builds on DeepSeek-V2, incorporating Multi-Head Latent Attention (MLA) and DeepSeekMoE for faster inference and lower training costs. The model has 671 billion parameters, but it only activates 37 billion at a time. This selective activation reduces computing demands while maintaining strong performance.

Unlike traditional MoE models that use auxiliary losses to balance expert usage, DeepSeek-V3 relies on dynamic bias adjustment. This method ensures experts are evenly utilized without reducing performance.
The model also features Multi-Token Prediction (MTP), allowing it to predict multiple tokens simultaneously. This improves training efficiency and enhances performance on complex tasks.
For example, if a user asks a coding-related question, DeepSeek-V3 activates experts specialized in programming while keeping others inactive. This targeted activation makes the model both powerful and resource-efficient.
2. Architectural Differences Between DeepSeek R1 and R1-Zero
DeepSeek R1 and DeepSeek R1-Zero benefit from the MoE framework but diverge in their implementation.
DeepSeek R1
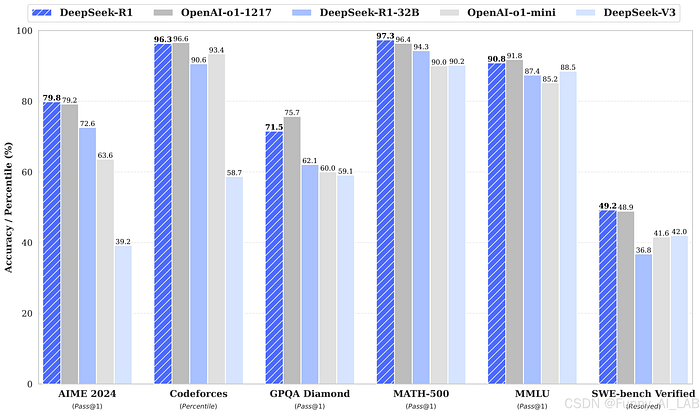
Employs full MoE capabilities while dynamically activating experts based on query complexity.
Uses reinforcement learning (RL) and supervised fine-tuning for better readability and logical consistency.
Incorporates load balancing strategies to ensure no single expert becomes overwhelmed.
DeepSeek R1-Zero
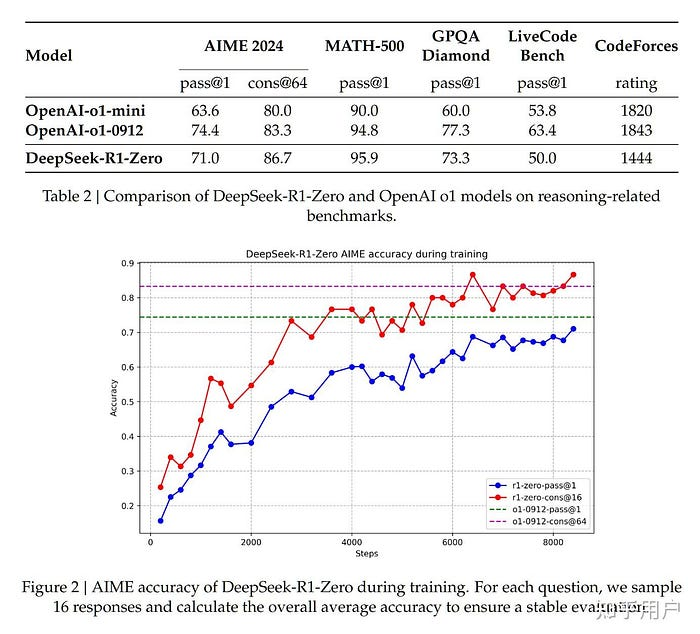
Uses a similar MoE structure but prioritizes zero-shot generalization rather than fine-tuned task adaptation.
Operates solely through reinforcement learning, optimizing its ability to tackle unseen tasks.
Exhibits lower initial accuracy but improves over time through self-learning.
Training Methodology: How DeepSeek Models Learn
DeepSeek R1 and DeepSeek R1-Zero use advanced training methods to improve the learning of large language models (LLMs). Both models apply innovative techniques to boost reasoning skills, but they follow different training approaches.
1. DeepSeek R1: Hybrid Training Approach
DeepSeek R1 follows a multi-phase training process, combining reinforcement learning with supervised fine-tuning for maximum reasoning ability.
Training Phases:
Cold Start Phase: The model first fine-tunes on a small, high-quality dataset created from DeepSeek R1-Zero’s outputs. This step ensures clear and coherent responses from the start.
Reasoning Reinforcement Learning Phase: Large-scale RL improves the model’s reasoning skills across different tasks.
Rejection Sampling and Fine-Tuning Phase: The model generates multiple responses, keeps only the correct and readable ones, and then undergoes further fine-tuning.
Diverse Reinforcement Learning Phase: The model trains on a variety of tasks, using rule-based rewards for structured problems like math and LLM feedback for other areas.

2. DeepSeek R1-Zero: Pure Reinforcement Learning
DeepSeek R1-Zero relies entirely on reinforcement learning, eliminating the need for supervised training data.
Key Training Techniques:
Reinforcement Learning Only: It learns entirely through reinforcement learning, using a method called Group Relative Policy Optimization (GRPO), which simplifies the process by removing the need for critical networks.
Rule-Based Rewards: It follows predefined rules to calculate rewards based on accuracy and response format. This approach reduces resource use while still delivering strong performance on various benchmarks.
Exploration-Driven Sampling: It explores different learning paths to adapt to new scenarios, leading to improved reasoning skills.

Overview of Training Efficiency and Resource Requirements
DeepSeek R1
Resource Requirements: It needs more computing power because it follows a multi-phase training process, combining supervised and reinforcement learning (RL). This extra effort improves output readability and coherence.
Training Efficiency: Although it consumes more resources, its use of high-quality datasets in the early stages (cold-start phase) lays a strong foundation, making later RL training more effective.
DeepSeek R1-Zero
Resource Requirements: It uses a more cost-effective approach, relying only on reinforcement learning. It uses rule-based rewards instead of complex critic models, which significantly lowers computing costs.
Training Efficiency: Despite being more straightforward, it performs well on benchmarks, proving that models can be trained effectively without extensive supervised fine-tuning. Its exploration-driven sampling also improves adaptability while keeping costs low.
BenchmarkDeepSeek R1DeepSeek R1-Zero
AIME 2024 (Pass@1)79.8% (Surpasses OpenAI’s o1-1217)15.6% → 71.0% (After training)
MATH-50097.3% (Matches OpenAI models)95.9% (Close performance)
GPQA Diamond71.5%73.3%
CodeForces (Elo)2029 (Beats 96.3% of humans)Struggles in coding tasks
DeepSeek R1 excels in reasoning-intensive tasks, while R1-Zero improves over time but starts with lower accuracy.
How to Use DeepSeek Models with Hugging Face and APIs
You can run DeepSeek models (DeepSeek-V3, DeepSeek-R1, and DeepSeek-R1-Zero) using Hugging Face and API calls. Follow these steps to set up and run them.
1. Running DeepSeek-V3
Step 1: Clone the Repository
Run the following commands to download the DeepSeek-V3 repository and install the required dependencies:
git clone https://github.com/deepseek-ai/DeepSeek-V3.git
cd DeepSeek-V3/inference
pip install -r requirements.txt
Step 2: Download Model Weights
You can download the model weights from Hugging Face. Replace with DeepSeek-V3 or DeepSeek-V3-Base:
huggingface-cli repo download –revision main –local-dir /path/to/DeepSeek-V3
Move the downloaded weights to /path/to/DeepSeek-V3.
Step 3: Convert Model Weights
Run the following command to convert the model weights:
python convert.py –hf-ckpt-path /path/to/DeepSeek-V3 –save-path /path/to/DeepSeek-V3-Demo –n-experts 256 –model-parallel 16
Step 4: Run Inference
Use this command to interact with the model in real-time:
torchrun –nnodes 2 –nproc-per-node 8 generate.py –node-rank $RANK –master-addr $ADDR –ckpt-path /path/to/DeepSeek-V3-Demo –config configs/config_671B.json –interactive –temperature 0.7 –max-new-tokens 200
2. Running DeepSeek-R1
Step 1: Install and Run the Model
Install Ollama and run DeepSeek-R1:
ollama run deepseek-r1:14b
Step 2: Create a Python Script
Create a file called test.py and add the following code:
import ollama
model_name = ‘deepseek-r1:14b’
question = ‘How to solve a quadratic equation x^2 + 5*x + 6 = 0’
response = ollama.chat(model=model_name, messages=[
{‘role’: ‘user’, ‘content’: question},
])
answer = response[‘message’][‘content’]
print(answer)
with open(“OutputOllama.txt”, “w”, encoding=“utf-8”) as file:
file.write(answer)
Step 3: Run the Script
Ensure Ollama is installed, then run:
pip install ollama
python test.py
3. Running DeepSeek-R1-Zero
Step 1: Install Required Libraries
Install the OpenAI library to use the DeepSeek API:
pip install openai
Step 2: Create a Python Script
Create a file called deepseek_r1_zero.py and add the following code:
from openai import OpenAI
client = OpenAI(api_key=“”, base_url=“https://api.deepseek.com”)
messages = [{“role”: “user”, “content”: “What is the capital of France?”}]
response = client.chat.completions.create(
model=“deepseek-r1-zero”,
messages=messages
)
content = response.choices[0].message.content
print(“Answer:”, content)
messages.append({‘role’: ‘assistant’, ‘content’: content})
messages.append({‘role’: ‘user’, ‘content’: “Can you explain why?”})
response = client.chat.completions.create(
model=“deepseek-r1-zero”,
messages=messages
)
content = response.choices[0].message.content
print(“Explanation:”, content)
Step 3: Run the Script
Replace with your actual API key, then run:
python deepseek_r1_zero.py
You can easily set up and run DeepSeek models for different AI tasks!
Final Thoughts
DeepSeek’s latest models—V3, R1, and R1-Zero—bring significant advancements in AI reasoning, NLP, and reinforcement learning. DeepSeek R1 dominates structured reasoning tasks, V3 offers broad NLP capabilities, and R1-Zero showcases innovative self-learning potential.
With growing adoption, these models will shape AI applications across education, finance, healthcare, and legal tech.